In a groundbreaking development, researchers have introduced Ceograph, a cutting-edge artificial intelligence model designed to predict cancer outcomes accurately by analyzing tissue samples. Published in the journal Nature Communications, this innovative approach harnesses the power of AI to decode the spatial arrangement of cells within tissue samples, providing crucial insights into the likely course of the disease and enabling personalized treatment strategies.
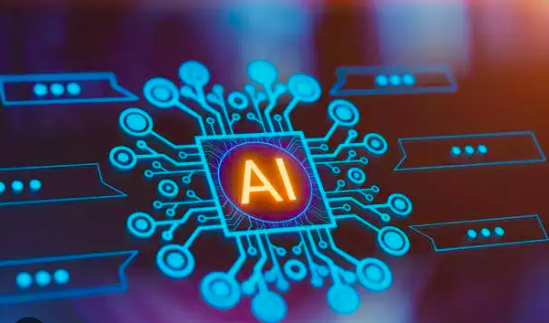
The analogy of cell spatial organization as a complex jigsaw puzzle, where each cell serves as a unique piece fitting meticulously together to form a cohesive tissue or organ structure, underscores the complexity unveiled by Ceograph. According to study leader Professor Guanghua Xiao from the University of Texas Southwestern Medical Center, the study showcases AI’s remarkable ability to grasp intricate spatial relationships among cells, extracting subtle information previously beyond human comprehension while predicting patient outcomes.
Traditionally, pathologists interpret tissue samples collected from patients and placed on slides for diagnosis. However, this manual process is time-consuming, subject to interpretation variations among pathologists, and may overlook subtle features crucial for understanding a patient’s condition.
Previous AI models focused on some aspects of a pathologist’s job, such as identifying cell types or using cell proximity as a proxy for interactions. However, these models fell short in replicating complex aspects of tissue image interpretation, like discerning patterns in cell spatial organization and eliminating extraneous “noise” that could hinder accurate analyses.
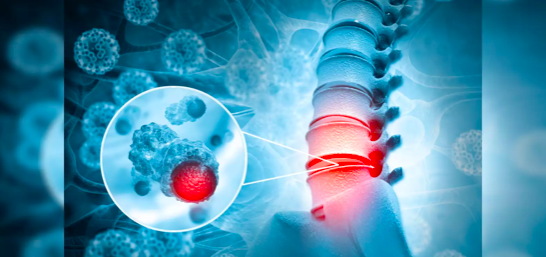
Ceograph, however, mimics how pathologists read tissue slides, starting with the detection of cells in images and their positions. It goes further by identifying cell types, morphology, and spatial distribution, creating a comprehensive map for analyzing the arrangement, distribution, and interactions of cells.
The researchers successfully applied Ceograph to three clinical scenarios using pathology slides. It excelled in distinguishing between subtypes of lung cancer, predicting the likelihood of malignant oral disorders progressing to cancer, and identifying lung cancer patients likely to respond to specific medications.
Ceograph’s superior performance in predicting patient outcomes underscores its potential to revolutionize pathology analyses, offering a more efficient and accurate alternative to traditional methods. Professor Xiao emphasizes the interpretability of Ceograph’s features, providing valuable biological insights into how changes in cell-cell spatial interactions could lead to diverse functional consequences.
These findings mark a significant stride in the integration of AI into medical care, presenting an opportunity to enhance the efficiency and accuracy of pathology analyses. Ceograph holds the promise of streamlining targeted preventive measures for high-risk populations and optimizing treatment selection for individual patients, heralding a new era in cancer prediction and personalized medicine.
To register for our next masterclass please click hereĀ https://linktr.ee/docpreneur